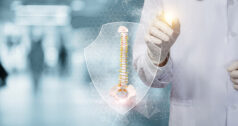
There’s little doubt that big data is shaping the future of patient care and Kevin McGann, President & CEO of Accelus, believes capturing reams of clinical information will play a pivotal role in the advancement of spine surgery.
“Analyzing vast amounts of patient data can assist surgeons in making more informed decisions and tailoring treatments to individual patients,” he said. “This will contribute to better outcomes and the delivery of value-based care, ensuring that surgeries are effective and cost-efficient.”
However, questions remain about the ways that captured clinical information will be applied to spine surgery. A predictive model powered by artificial intelligence (AI) and machine learning (ML) developed by researchers at Washington University’s AI for Health Institute provides some clarity.
Capitalizing on Clinical Data
Chenyang Lu, Ph.D., the Fullgraf Professor at Washington University’s McKelvey School of Engineering, collaborated with Jacob Greenberg, M.D., Assistant Professor of Neurosurgery at Washington University’s School of Medicine, to establish a way to more accurately predict recovery from lumbar spine surgery based on the characteristics of individual patients.
Dr. Lu is focused on increasing the applications of advanced AI to solve complex medical problems. “AI has become an important tool in data-driven care,” he said. “Combining it with machine learning and a ton of data can predict clinical outcomes.”
His lab’s staff works on a wide range of important clinical problems that require data-driven, AI-based solutions. The advanced AI models that they develop can be seamlessly integrated into medicine to impact patient care, Dr. Lu said.
Researchers have previously used data captured from Fitbit wearable devices to measure activity levels during surgical patients’ recoveries and compare the activity levels over time. However, measuring activity levels alone wasn’t enough to predict how well patients do after surgery. Longitudinal data assessment creates a more accurate picture and recent developments in smartphone communication apps and wearable technologies allow care teams to capture data on patient conditions before and after surgery.
In previous research, Dr. Lu’s team showed that patient-reported outcomes combined with objective data captured by wearable devices improved the accuracy of recovery predictions compared to traditional post-op patient assessments. During that initial study, which was published in the journal Neurosurgery, the researchers correlated Fitbit data with numerous ecological momentary assessments (EMAs) — prompts sent to patients’ smartphones multiple times throughout the day, reminding them to answer survey questions about their mood, pain levels and behavior.
The researchers combined data captured by wearables, the EMAs and clinical records to gather a broad range of information about the patients: clinical characteristics, physical activities, subjective reports of pain and mental health. They then developed an advanced statistical tool to analyze the complex data.
Their current work built off that baseline concept with a new ML learning technique that predicts multiple recovery outcomes. The model takes shared information on interrelated tasks of predicting different outcomes and leverages the shared information to understand ways to make a more accurate prediction. It then produces a predicted change for each patient’s postoperative pain interference and physical function score.
The findings of the predictive model are published in the journal Proceedings of the ACM on Interactive, Mobile, Wearable and Ubiquitous Technologies.
Solving a Complex Issue
Ziqi Xu, a Ph.D. student at Washington University and member of Dr. Lu’s research team, said predicting outcomes before spine surgery can establish realistic expectations and help with early interventions and identifying high-risk factors. He believes ML could replace traditional patient questionnaires, which fail to capture the long-term dynamics of a patient’s physical and psychological patterns and don’t assess the complexity of surgical recovery.
Determining how well a patient will recover from spine surgery is a complicated puzzle that’s influenced by physical and mental health. Worrying about the recovery process and physiological factors can exacerbate post-op pain.
Surgeons who are aware of these factors before surgery can create personalized treatment plans to improve the postoperative experience for individual patients. The ML model developed by Dr. Lu’s team provides a more accurate big-picture look at the multiple factors that impact a patient’s recovery from spine surgery.
The researchers said predicting outcomes for patients who undergo surgery in the lumbar spine is especially important because results can vary greatly. They added that relying on patient-reported outcomes doesn’t capture the dynamics of patients’ conditions before surgery and the ways the conditions impact recoveries.
“Our results also demonstrate the complementary nature of the multi-modal data and their contributions to each outcome through model interpretation,” they noted. “By integrating clinical data and multi-modal mobile sensing technology, our work represents a promising step toward predicting comprehensive surgical outcomes and approaching personalized surgery.”
Dr. Greenberg said the study is ongoing as the researchers continue to fine-tune their models so they can take more detailed assessments, predict outcomes and understand what types of factors can potentially be modified to improve longer-term outcomes.
DC
Dan Cook is a Senior Editor at ORTHOWORLD. He develops content focused on important industry trends, top thought leaders and innovative technologies.