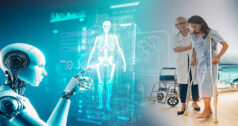
A standing-room-only crowd packed a large session hall for the President’s Symposium at this year’s American Academy of Orthopaedic Surgeons Annual Meeting to learn about the ways that artificial intelligence (AI) and machine learning (ML) could impact the future of orthopedic care.
The panel discussion raised more questions than answers, but left no doubt that the technologies will play important roles in advancing patient outcomes. A team of researchers at Wake Forest University School of Medicine attempted to quantify the ways ML could improve the treatment of patients recovering from lower extremity fractures and published their findings in the Journal of Orthopaedic Research.
The researchers combined post-injury gait analysis with ML models to create a predictive tool for assessing complications such as infection, malunion and hardware irritation in 681 patients. The study’s participants underwent gait analysis with a chest-mounted inertial measurement unit device. ML models processed the data, emphasizing 12 essential gait variables. The analysis revealed a notable negative correlation between the time elapsed from injury to the initial gait analysis and recovery outcomes, suggesting that delays in performing the initial gait analysis are associated with a higher risk of complications.
ML was able to accurately predict which patients were at increased risk of suffering complications during their recoveries. “This advancement is a significant stride toward personalized and predictive orthopedic medicine,” the researchers wrote. “The findings underscore a shift toward a data-informed, proactive approach in orthopedics, enhancing patient outcomes through early detection and intervention.”
Their study suggests that ML can fill a gap in the current understanding of orthopedic recovery protocols and provide a foundation for improved patient care strategies.
Predicting Best Outcomes
The research team was drawn to this line of research by the challenge posed by standardized fracture recovery protocols and the pressing need for improved patient-specific treatment paradigms.
“With advances in machine learning and biomechanical monitoring, we identified a unique opportunity to synergistically apply these technologies to predict recovery outcomes,” said Mostafa Rezapour, Ph.D., Research Fellow at the Center for Artificial Intelligence Research of Wake Forest University School of Medicine and the study’s lead author. “We were motivated by the potential of unraveling complex patterns in gait analysis data that are not readily apparent to human observers, thereby enabling precision medicine approaches that could drastically enhance the efficiency and effectiveness of orthopedic rehabilitation.”
Dr. Rezapour said integrating ML with real-time gait analysis data can significantly elevate the predictive capabilities in orthopedic care, specifically in monitoring and predicting the course of fracture recovery.
Orthopedic caregivers ultimately want to return patients to their pre-injury functional levels, the researchers noted. They also acknowledged the difficulty in managing patients’ rehabilitation, especially in patients who suffered lower extremity fractures.
Rehab for these patients typically focuses on early ambulation, improved range of motion and careful weight-bearing to prevent harm to the fracture site and surrounding soft tissues. Weight-bearing protocols depend on the fracture’s stability and fixation approach, the researchers noted. They said effective weight-bearing guidelines require a blend of clinical expertise and clinical evidence, and added that patient non-compliance with prescribed rehab programs, pain and mobility challenges are potential barriers to ensuring patients fully recover from their injuries.
“There’s a scarcity of data on recovery rates and returning to preinjury functionality following lower extremity fractures,” the researchers wrote. “This is where gait analysis becomes invaluable, offering insights into a patient’s rehabilitation journey.”
The researchers were surprised by the high degree of predictability of specific gait parameters on postoperative complications and the overall recovery trajectory. “That was notable, especially in the context of early rehabilitation phases, where traditional assessments might not fully capture the nuances that machine learning can discern,” Dr. Rezapour said. “This suggests that machine learning not only has a place in clinical settings but could redefine standard monitoring protocols.”
A Model Approach
The researchers said their research is novel and to their knowledge, the first time that predictive models have been applied to orthopedic complications. Dr. Rezapour believes the study’s findings support the data-driven care movement in orthopedics by providing a clear demonstration of how ML models, developed from gait analysis data, can enhance predictive accuracy and clinical decision-making.
“We’ve shown that these models can identify risk thresholds for individual patients, predict potential complications and suggest optimal rehabilitation strategies tailored to specific recovery profiles,” he said. “By transitioning from a one-size-fits-all approach to more personalized treatment and rehabilitation plans, our research aligns closely with the broader objectives of precision medicine in orthopedics.”
Patient care, data analysis and ML are poised to play a pivotal role in orthopedics by serving as the backbone for decision support systems that can handle complex datasets to deliver actionable insights, according to Dr. Rezapour.
“Beyond predictive analytics, I foresee these technologies facilitating continuous monitoring and adaptive interventions that are dynamically tailored to patient progress,” he said. “As these tools evolve, they will likely become integral to developing proactive treatment modalities, optimizing resource allocation and ultimately improving the standards and outcomes of patient care through data-driven insights.”
Dr. Rezapour said current limitations of AI and ML in orthopedics include data quality and quantity challenges, the need for robust, interpretable models that clinicians can trust and the integration of AI tools into existing healthcare systems.
“Addressing these barriers to adoption requires multidisciplinary collaboration to ensure AI and machine learning are developed and validated rigorously, fit seamlessly into clinical workflows and are accompanied by training programs for healthcare professionals,” Dr. Rezapour said. “Enhancing data security and patient privacy is also crucial to fostering trust and acceptance among users of the technologies.”
He believes that overcoming these barriers will be key to realizing the full potential of AI and ML in enhancing orthopedic care.
Dr. Rezapour made a point to acknowledge his research colleagues and said their collaboration serves as a model of how experts in distinct fields can work together to merge cutting-edge AI with clinical expertise to push the boundaries of medical science.
“Such partnerships are vital for the advancement of integrated patient care, and our successful collaboration highlights the significant potential of interdisciplinary efforts in enhancing research and clinical outcomes,” he said.
DC
Dan Cook is a Senior Editor at ORTHOWORLD. He develops content focused on important industry trends, top thought leaders and innovative technologies.